
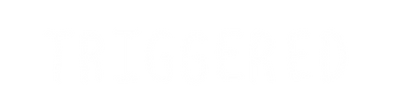
using human-AI dialogue for problem understanding in collaborative design


This project shows my individual research project, executed as part of my honours master program.
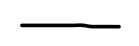
Supervisor
Prof. Dr. Peter Lloyd
Dr. Senthil Chandrasegaran
Vera van der Burg
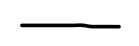
Collab
TU Delft
Design Intelligence Lab
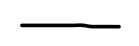
Details
Honours Master Research
3 Semester duration
At TU Delft/ DI-Lab
' I repeatedly see people dropping their plastic waste right next to the trash cans. I think we should investigate that behaviour
more closely'
' I am not sure. I believe that the big factories around the city are the main reason for pollution. We should have a look at their businesses!'

Creative conversation among designers and stakeholders in a
design project enables new ideas to naturally originate and evolve. Language allows for the exchange of values, priorities, and past experience whilst keeping solution forms usefully ambiguous. Yet there is a danger that only the language of people directly involved in the design process gets to be heard, limiting how inclusively the problems are interpreted, which in turn can impede how complex design problems are addressed.

Recent advances in artificial intelligence (AI) have shown the exclusionary spaces that are often inhabited by designers, engineers, and developers of new artefacts and technologies. On the other hand, text data used to train language models for machine learning applications have the potential to highlight societal biases in ways that designers can utilise. In this paper, we report the results of an exploratory study using AI text generation to synthesize and narrate opinions and experiences that may be unfamiliar to designers.

Research Question
How can AI -specifically a text generator powered by a language model- be used to expose and counter limitations in early-stage design processes by bringing in an alternative voice into design conversation?

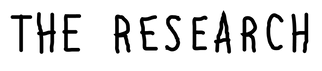
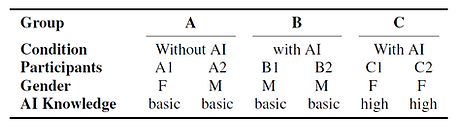
To answer our research questions I conducted an exploratory study in which designers interact with a textual AI system when working on a complex socio-technical problem. The exploratory study featured six voluntary participants, all students or researchers in design (4 female, 2 male) in a European technical university. They were split into three pairs of designers (see table on the left) to work together on the early phases of the problem brief. One pair (Group A) was assigned to a baseline condition without access to the AI interface. The two remaining pairs of designers (Groups B &C) had access to an AI text generation interface powered by a language model.
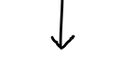
The Study Brief
The design brief given to participants was split into three steps.
Step 1–Introduction. Participants were introduced to the problem through an executive summary of a 2014 report on street harassment in the United States. They were encouraged to note down interesting and inspiring insights as starting points for the later investigation.
Step 2–Main Investigation. Next, participants were asked to explore the problem of harassment in public spaces, starting with the notes taken during the introduction, and write down further thoughts, ideas, and questions that emerged in the exploration.
Step 3–Framing. In the last part of the study, participants were asked to reflect on their design process and summarise their understanding of the problem in a short statement.
The brief for the designers was to explore the problem of harassment of women in public spaces and suggest ways in which instances of harassment could be reduced. The problem is obviously gendered with the potential for strong views and importantly the solution form is not assumed.
problem brief for participants
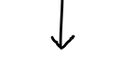
The Text Generator
For the study I used Inferkit, an online text generator, powered by a language model. The interface is simple, the user can give a text prompt and click 'generate text'. A few adjustments about style and probability of the model can be made on the left.
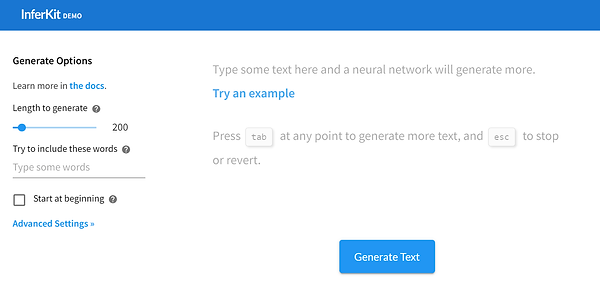

We used linkography as a method to uncover links between different ‘design moves’—decisions or actions that changed the problem, solution, or the state of understanding of a design team. Adapting the method to visualize the interaction between AI and designers, we categorise design moves into two kinds: a thought, idea, or step in the process by a human that changes the situation and/or expands the understanding of a problem (black nodes in the figures below), and (b) a response given by the AI text generator based on a text prompt entered by the human participant (green nodes). A design move can be made in three ways: during a conversation between designers; from an AI in response to an input by the designer; or as a ‘clarification’ by the AI based on already generated text (see Figure below).

Here an excerpt from Group B's interaction, exemplifying how ‘design moves’ evolve in a conversation between designers and AI. The moves involve conversations between participants—indicated in the format ‘Participant: [spoken dialogue]’—and interactions between a participant and the AI—indicated by the format ‘Input by Participant: [written dialogue]’ or ‘Output by AI: [text output].’ Moves to which the designers are primary contributors are indicated by black nodes, while moves to which the AI is the contributor are coloured green. Differences between direct (continuous lines) and indirect (dotted lines) are illustrated to show different possible AI-design-move evolutions.
A sample of of design moves from Group B showing the interpretation of moves based on participants’ conversations with each other, and responses from and to the AI. A sample of the actual transcript and how it is interpreted is provided in the figure below.
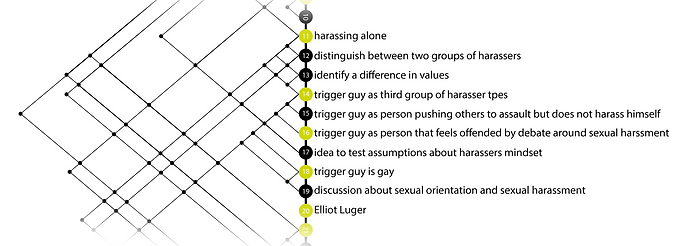

Linkographs
In the linkographs we produced, we identified three structures that have been established to be indicative of certain behaviours in designing: (a) ‘critical moves’ are moves that have many links to preceding design moves—called ‘backlinks’ indicating a convergence of previously explored ideas—or to subsequent
design moves—called ‘forelinks’, indicating a source of divergent thinking. (b) ‘chunks’ represent a sequence of design moves that are highly interconnected, forming a triangular cluster of nodes. Chunks indicate a close examination of the problem. (c) A ‘web’ is a smaller and denser version of a chunk, showing an intense burst of investigation or idea generation. Processes that show chunks and webs have been found to be more productive and creative than processes that do not.
the control group (Group A) has the least design moves (22) as opposed to Group B (31 moves) and C (30 moves). While a chunk (moves 6–10) and a web (moves 14–17) exist in Group A, they are relatively small and do not result in ideas taken forward to the final framing. There are also no critical moves that form forelinks in Group A, though a few penultimate moves are indeed critical, with backlinks to moves leading up to the first one. Examining the transcript, we see that some initial
ideas that were explored at the start informed the final framing of the problem. This is also indicative of a form of fixation that is observed among expert designers, an inability to discard initial concepts and problem frames
Group A - Baseline Condition
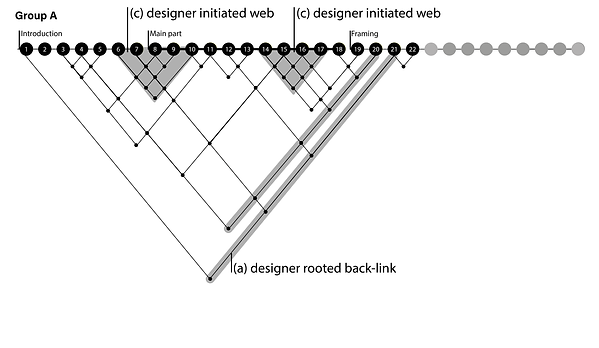

Group B shows two critical moves that are forelinks (3 and 14), indicating divergent thinking stemming from
those moves. Of these, move 14 is one that is initiated by the AI stimulus, which is discussed later in this section. The web and chunk in Group B are comparable in size to Group A, but the end of the web is also the critical move mentioned above, indicating that the intense discussion led to a fruitful and divergent critical move.
Group B
Group C shows a large chunk that is initiated by a
stimulus from the AI (move 12), initiating a discussion that forms several links to the two critical moves in the linkograph. This indicates that while a single move did not spark divergent thinking, intense discussion around the AI-initiated move influenced the progression of ideas and the final framing of the problem.
Group C

Interaction Dynamics
Examining the actual interactions (conversations, text inputs, and outputs from the text generator) allows us to characterise the different ways in which the participants work with the AI. A timeline of design moves for the two groups that used the AI text generator is taken from the Linkographs. Each design move is shown as a numbered node in the figure. Black design moves primarily contain contributions by designers while green design moves contain contributions by the AI. Moves 7–26 in Group B are indicative of back and forth switches between the designer and the AI. We term these “alternating conversations.” Another pattern is seen in Group C in moves 10–12 where short investigations using AI, leading to long conversations (moves 13–23) among designers. We term this set of moves as “enhancing conversation,” indicating the
contribution of AI to the conversation.
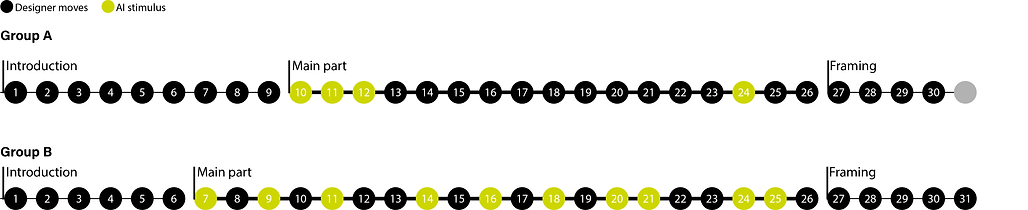
Perceived Roles
It was observed, that designers assigned different roles to the text generator. These roles were grouped and are presented on the left.
We found designers to mostly use the text output as a inspiration stimulus. In these scenarios the tool was used to enhance conversations by receiving related input. Designers discovered new aspects of the ideas or problems they were discussing.
The Inspirator
‚Who is the trigger guy? Probably the person pushing the other people to do it. [...] this person, him or herself isn‘t doing anything.‘
‚So the person that wants to cross boundaries, but it‘s kind of afraid of doing it themselves [...].‘
‚I will generate some more text.‘
Conversation between participants
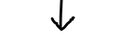
Conversation with AI
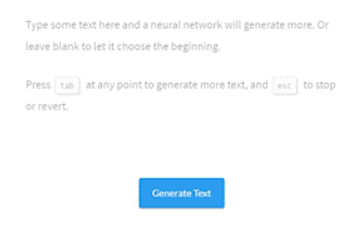
In a second scenario the designers ‘asked the AI for its opinion’, by feeding in their ideas, thoughts and assumptions.
The Critique
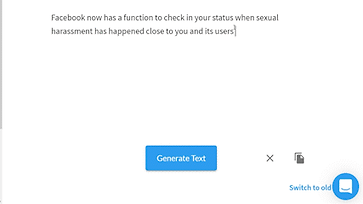
‚Because you might be wrongfully accusing somebody?‘
‚I know that in the U.S., there were some issues with the police and universities covering up a lot of stuff, actually kicking out females for going through with the.
Conversation between participants
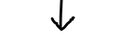
Conversation with AI
We could also categorise AI as playing a mediating
role. “It is like someone telling us a story”, a participant
observed. The participants used the tool to create awareness, test their own assumptions, and provide a change in perspective. One
group used the tool to better understand the mindset of harassers and their way of reasoning
The Mediator

Conversation between participants
Conversation with AI
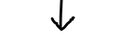
‚We cannot empathize with the harraser, so we don‘t know the mindset.‘

Based on the results stated, we want to make the argument that AI can be potentially useful as a research assistant in these early open-ended problem explorations. We have shown how AI suggestions can aid divergent thinking by helping designers to think of new problem dimensions and explore the unforeseen consequences of proposed solutions. This can both mitigate against design fixation and allow designers to consider more nuanced aspects of the design brief. In this sense a conversation with AI could be seen as getting beyond the rationality of the designers, taking them to areas of the problem that they would not intuitively go to given their preconceptions and natural biases.
AI against fixation
the main mechanics behind
Repetition
Recent work looking at problem framing in the form of unusual short phrases has shown that distinctive concepts expressed in language can frame design problem solving when repeated. We saw this in our study through a suggestion made by the AI (‘trigger guy’). The repetition of this unusual phrase indicates that it became a problem frame for the designers and this was backed up by the ‘forelink’ structure of the corresponding linkograph. The potential for AI to come up with unusual phrases is obviously considerable, and can help frame explorations in an abductive way (i.e. the designers adapting reasons to fit the idea rather than working deductively). How effective these unusual phrases are in stimulating exploration could be influenced by the training data, a possible future area of study.
Storytelling
We noted the ability of the AI to create mini stories that appeared to foster a deeper emotional connection between the designers and the AI as important mechanism. Storytelling has been identified as a way of articulating experience in designing, particularly in communicating conflicting values. The fact that AI comes without moral self-censorship can lead to interesting and confronting interactions where alternative and oppositional stances appear to be taken. Story ‘structures’ remain an interesting area of exploration for designer/AI collaboration and, as we noted earlier, is one of the more advanced forms of creative practice using AI.
Roles